During Eye International Conference on May 29th, I presented a series of experiments on archive remixing, posing the question, “Is reimagining the new remix”?
Remixing has been, for the past decade, an important strategy used by artists and archive specialists to address the challenge of making visual and audiovisual archives accessible to an audience beyond researchers. From a curatorial perspective, remixing infuses old archives with new authorship, as they become ingredients in an artist’s orchestration. Examples of these entanglements can be widely found in the work of experimental filmmakers working with old materials in a sub-genre known as found footage films, such as the work of Peter Tscherkassky and Gustav Deutsch, and more recent visual artists like Rodell Warner’s “Augmented Archive” series (2019-2024) and Aimée Zito Lema’s “214322” (2019).
In the experiments I presented during the conference, part of a loosely tied series I call “Typologies of Delusion,” I propose using generative artificial intelligence (GenAI) as a set of tools to appropriate audiovisual archives in a slightly different fashion than remixing. I call it “reimagining”.
While remixing operates under the logic of sampling, which consists of picking fragments from an original piece and rearranging them in a new piece, generative AI tools operate under a different logic: the logic of reimagination. The key difference between the two, I claim, is that remixing appropriates archives through their perceived media, while reimagining appropriates them through their data.
Reimagining involves using a media piece’s perceptual features and metadata to feed an AI model. Whether this data is used for training or as a reference, the result will resemble some aesthetic dimensions of the original piece. However, these resemblances will not contain samples of the original media. Unlike remixes, these images will be literally reimagined.
Flaws
In two previous experiments of this series, titled “Typologies 01: Faces” and “Typologies 02: Film Stills” (which I’ve also written about on this website), I attempted to reimagine archives using the Polygoon Journaal collection. I selected approximately 100 images to train LoRA models compatible with Stable Diffusion, aiming to reveal unexplored imagery within the collection. This approach was based on the supposition that generative AI operates like a Jungian visual unconscious, composed of countless images. Theoretically, I thought the model’s output should extend that visual unconscious.
I hypothesised that if this unconscious were populated by images from one coherent and specific collection, like the Polygoon Journaal, the resulting pictures would reveal the collection’s biases and, consequently, a synthetic version of its gaze.
In retrospect, I acknowledge that these experiments were overly ambitious and arose from a naive understanding of generative AI. The output was fallacious. The models could replicate the visual style of the Polygoon but proved unreliable as a source for a better understanding of the collection, either from a historical or philosophical perspective. If these models were to capture something akin to a visual unconscious, that unconscious would be informed by the billions of images forming the model’s foundational dataset, not just those used to fine-tune the model, as I did with the LoRAs. A deeper reflection on this topic will be published in a book that Open Archief plans to release by the end of this year.
Despite their limitations, the Polygoon experiments were not in vain. While they demonstrated that reimagination, like remix practices, is intrinsically disconnected from an archive’s original context, they also suggest that generative AI has the potential to provide insights into an archive. These insights, however, are aesthetic rather than historical in nature.
Typology 03: Serpentines
For my third experiment using typologies as a visual method to compare and conceptualise the affordances of generative AI, I selected a short fragment from the Lumière brothers’ emblematic early cinema piece from 1896, “La Serpentine.” This film portrays a female figure (performed by Loie Fuller) dancing in a multilayered silk dress on what appears to be a windy stage. The character’s hypnotic twirls and motions are evocative of a snake, hence the title “La Serpentine.” I considered this piece ideal for demonstrating generative AI’s potential to reimagine, as it offered a wealth of shapes and movements that could open up a wide range of possible imagery to intertwine with the original.
The first step in my workflow was to isolate the dancer from the background, as she was the only element I intended to reimagine. Using a local AI tool integrated into DaVinci Resolve, my preferred video editor, I easily achieved this with just a few clicks.
Next, I employed ControlNet and AnimateDiff, two popular tools commonly used with Stable Diffusion generative models, to extract depth and shape features from each video frame.
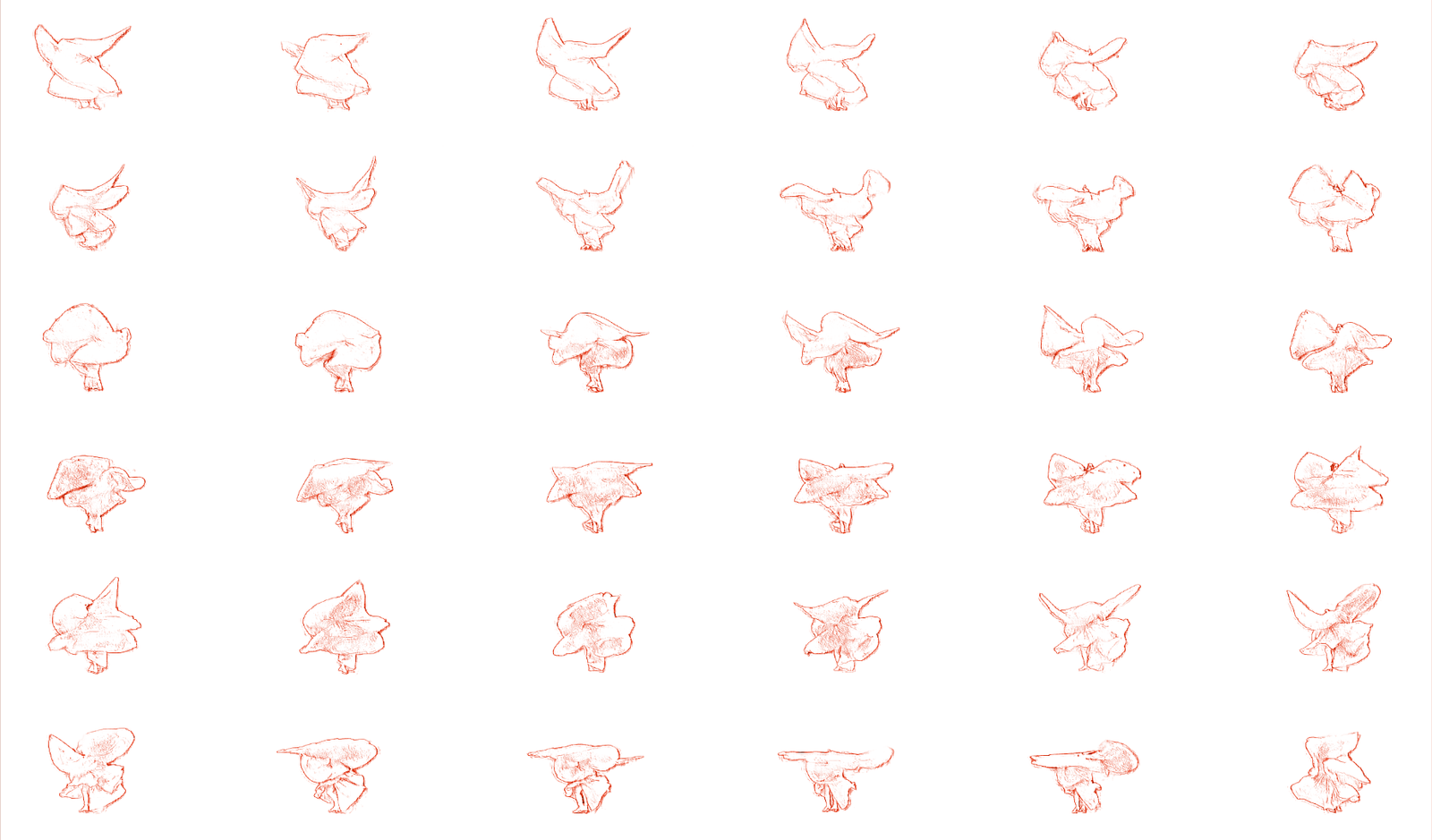
After processing this information, I input a series of prompts to reimagine the fragment using Stable Diffusion XL. Here is a selection of samples:
Affordances of focalised reimagination
This third visual typology, Serpentines, demonstrates the potential of generative AI to reimagine aspects of audiovisual heritage through the lens of a large diffusion model prompted to envision specific objects. Unlike the previous two typologies, Faces and Film Stills, my ambitions with this experiment are more focused and, in my view, more aligned with what this technology can realistically achieve today. The research question for the first two typologies was along the lines of “Can we use generative AI to synthesise and discover unexplored dimensions of an archive’s gaze?” For this typology, the question is, “Can we use generative AI to extrapolate visual features of archives to highlight – and eventually isolate – some of their aesthetic dimensions?”
The first research question deals with the contents and historiography of an archive. In contrast, the second focuses on applying generative AI to a long-developed research field in data visualisation, known as feature mapping.
Practical applications I foresee for this approach engage with the notion of generous interfaces as creative entry points to access and discover audiovisual heritage. To conclude this note, here are a few concrete examples:
- An art installation where several audiovisual pieces are disguised using generative AI. Visitors could discover the original pieces through an interactive and playful narrative. Additionally, with a relatively fast workflow to reimagine and render images, visitors could generate their own disguises of the originals, challenging others (e.g., friends) to guess the pieces.
- A dance choreography informed by reimagined selections of audiovisual archives. Generative AI could serve as a visualization tool to support the choreographer’s creative process. The reimaginations could also be used as audiovisual components in the final performance or as references for their creation.
- A data visualization methodology to compare isolated features of audiovisual heritage. Extracting and reimagining these features using the same prompt would follow the logic of defining a mathematical “Least Common Multiple” (LCM). Displaying multiple images using a unified style could help compare visual aspects of historical media from a sensorial perspective, such as facial gestures, body language, lens use (e.g., curvature and depth of field), or camera framing and angles.
I’m genuinely curious to hear your thoughts on this. Please feel free to share them in the comment section.
3 responses
Impressive work.
Have a blessed life.
Thanks. It is great to know that there’s interest in this experimental line of work in other parts of the world 🙏
Imaginary thinking, inspirational. Love it!
Miroslaw